- Home»
- Resources»
- Blog Posts»
- The Role of AI, Analytics, and Automation in Boosting Outpatient RCM Performance
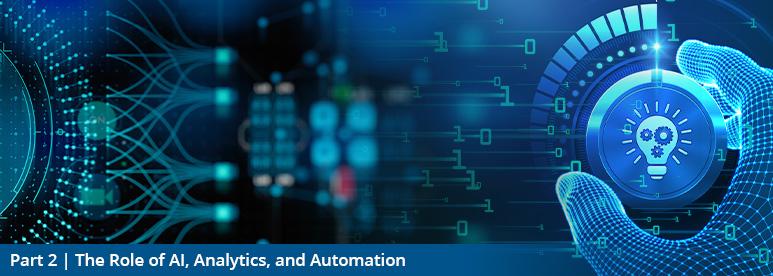
The Role of AI, Analytics, and Automation in Boosting Outpatient RCM Performance
November 10, 2022This blog is part of a series. Read Part 1 here.
We recently presented a webinar to the members of The Healthcare Financial Management Association (HFMA): Outpatient RCM Gap Analysis for a Better Bottom Line. In that webinar, we covered a variety of topics – from recent research findings to lessons learned from dozens of outpatient RCM implementations and two XiFin customer case studies. We summarized those topics in a previous blog post. Today, we’ll round out key webinar topics, exploring the role analytics, automation, and artificial intelligence (AI) play in Revenue Cycle Management (RCM) operational and financial performance.
Analytics-Informed RCM Workflow Optimization
Analytics drive insights into effective workflow automation. They provide a global understanding of an organization’s entire RCM process. They pinpoint the challenges. They unearth their root causes, illuminating the pathway to meaningful improvement.
The wide array of available analytics, however, can be as overwhelming as they are helpful – spanning clients and payors, ranging from ordering patterns to data quality. A set of analytical dashboards helps organize and prioritize data, allowing us not just to see trends, but also the drivers behind them. They enable comparisons among key performance indicators (KPIs), benchmarking of current against historical performance, and trend forecasting.
On a more granular level, analytics can help organizations understand payor behavior, which can have a significant impact on effective revenue cycle management. Payor reimbursement behavior is complicated and constantly changing. Payors introduce new products and plans. Providers move between in- and out-of-network status. Policies change. Service-level coverages are altered. These modifications manifest in billing as:
New denials
Changes in denial rate
Fluctuations in reimbursement percentages
Variations in time to payment
Failure to quickly recognize and adapt workflow to payor reimbursement behavior changes can result in costly appeals and increased write-offs.
Analytics drive early insight into changes in payor behavior. This “early warning system” helps organizations address root causes and modify workflows, as appropriate, to eliminate errors, reduce denials, and automate appeals management. A specific example involves a regular review of actual versus expected reimbursement, which is important to more accurately forecast revenue. It’s also critical to producing an accurate patient financial responsibility estimate.
Bridging the Gap Between Expected Payor Reimbursements and Patient Financial Responsibility Estimates
Patient responsibility estimates consider all relevant provider-specific pricing information, test or procedure information, and real-time eligibility. This process estimates the amount to collect from the patient at the time of service or at the time of order. It is predicated on receiving complete and accurate information back from the payor and having the appropriate expected reimbursement on record.
There are challenges, however, in managing expected reimbursements – and the associated data. Organizations have many contracted rates that demand accurate and timely maintenance. Non-contracted payors are another matter. It can be difficult to keep track of how much they will pay, by each CPT code – not to mention their denial rates. To complicate things further, many RCM systems use billed price to determine expected payment, which inflates expectations.
Analyzing recent reimbursement data helps mitigate these challenges. Applied machine learning can then produce expected fee schedules by payor and procedure codes, and these schedules can be regularly updated automatically, using the most recent data. The result: more accurate reimbursement predictions and, ultimately, more accurate patient financial responsibility estimates. And in turn, accurate patient out-of-pocket estimates are proven to reduce write-offs – while improving the patient experience.
The Direct Impact of Payor Behavior and Provider Engagement on Patient Experience
When RCM outpatient processes and teams ensure payor responsibilities are met – in combination with highly engaged ordering physicians and staff – the patient experience is elevated, and reimbursement maximized. Real-time analytics, however, are a necessity to support this approach, as are proactively managing expected pricing and addressing root causes through gap analysis and data exchange with physicians and patients. An adaptive, flexible, automated workflow also helps, especially when leveraging APIs that enable two-way digital conversations.
Enhancing and automating provider engagement can also make a difference in patient experience. To empower that, organizations need RCM systems that:
- Help minimize paper and manual processes
- Collect missing or incorrect information electronically and automatically
- Request clinical documentation up-front based on payor behavior insight (business-logic driven)
Analytics, automation, and AI can help yield tangible, meaningful improvements in RCM performance. To gain these data-driven insights and workflow efficiencies, RCM leaders need to advocate for an outpatient RCM solution that:
- Leverages a diverse set of APIs / interfaces to enhance interoperability with other systems
- Offers accurate patient responsibility estimation
- Is highly configurable (e.g., by procedure type, payor, payor plan) without IT involvement
- Allows overrides (e.g., for payor address and reason code)
- Automates denials and appeals management, including payor-specific forms and letters
- Sets automation actions and assignment of claims to users by expertise or effectiveness
For more detail about the role of AI, analytics, and automation in boosting outpatient RCM performance, access the webinar here.