- Home»
- Resources»
- Blog Posts»
- Practical Applications of Artificial Intelligence (AI) in Revenue Cycle Management
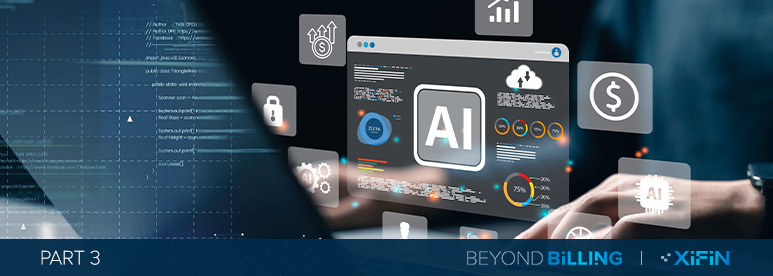
Practical Applications of Artificial Intelligence (AI) in Revenue Cycle Management
January 26, 2024In our first two blogs in this series, we explored the foundational terms and definitions to help understand what AI can do to solve real-world revenue cycle management (RCM) challenges and how everything AI-related starts with data. In this final post in the series, we’ll illustrate several practical examples of AI applied to make the RCM process faster, more cost-effective, and more patient- and physician-friendly. In the first real-world example, AI is deployed in the RCM process to remove friction from the patient’s experience. Requesting multiple pieces of information from patients creates friction and, therefore, points of abandonment. AI applied at the front end of the RCM process minimizes the amount of information requested from a patient to move forward in the RCM process.
Improving the Patient Experience with AI
One of the challenges labs and diagnostic service providers face is ensuring that the correct third-party insurance information for patients is captured. This is essential to process claims and receive reimbursement. This can be incredibly challenging in diagnostics as the provider may not see the patient and cannot collect the payor and plan information from the patient directly. This creates a point of friction.
The diagnostic provider doesn’t want to contact the ordering physician or the patient but sometimes must. Insurance information is complicated. A lot of data must be collected, which can be very cumbersome. It often requires a customer service agent to be on the phone with the patient to collect the information and it can be challenging for a patient to provide the precise payor plan details.
AI can help simplify this process for patients. The goal is to minimize the amount of insurance or demographic information requested from a patient so that the eligibility and benefits coverage part of the process can proceed. Using optical character recognition (OCR), an image of the front and back of a patient’s insurance card can be taken, the relevant text isolated, and used in an AI model to get the information needed to generate an eligibility request and confirm eligibility with that payor.
If taking an image of the insurance card isn’t possible, AI allows the patient to walk through a simplified online process and provide just a few pieces of data that allow the diagnostic provider to run eligibility verification and receive confirmed eligibility. For example, the patient can provide high-level payor information only, such as the name of the commercial payor or whether the coverage is Medicare or Medicaid, the state the patient resides in, and the subscriber ID, and AI can use this high-level data to get an eligibility response and confirm eligibility.
Once the eligibility response is received, the more detailed payor information can be presented back to the patient for confirmation. AI can map the eligibility response to the appropriate contract or payor plan within the RCM system.
Patient Responsibility Estimation
Now that the patient’s correct insurance information is captured, the workflow moves on to collect the patient’s financial responsibility payment. Calculating an accurate patient financial responsibility estimate is predicated on receiving complete and accurate information back from the payor and having the appropriate expected amount on record. The estimate must consider relevant provider-specific pricing information, test or procedure information, and real-time eligibility to determine patient responsibility amount.
The RCM system has accurate pricing information and now, based on the payor information capture process outlined above, also has:
- Detailed payor and plan information
- A real-time eligibility response
- Test or procedure information
This data is used to estimate patient financial responsibility. AI can also be used to determine if a claim is likely to be rejected because of incorrect or incomplete payor information or patient ineligibility, in which case automation can be applied to resolve most issues.
Negotiated Rates and Expect Pricing
Another way AI can help laboratories and diagnostic providers is in understanding and maintaining expected prices—what an organization can expect to collect from particular payors for particular procedures. For contracted payors, contracted rates are loaded into the RCM system. It’s important to track whether payors are paying those contracted rates and whether the organization is receiving the level of reimbursement expected. For non-contracted payors, it’s harder to know what the reimbursement rate will be. Historical data and AI can provide a good understanding of what can be expected.
AI can also be used to address and adapt to changes in ordering patterns, payor responses, and payor reimbursement behavior, as well as streamline denials and appeals management.
AI-Driven Workflow: Assigning Exceptions to be Worked by Billing Team Members
Another AI benefit relates to quickly determining the probability of reimbursement and assigning how claims are prioritized if a claim requires intervention that cannot be automated. With AI, these claims that require manual error processing (EP) are directed to the best available team member based on that team member’s past success with resolving that particular error type.
- Claim errors are prioritized by payment risk using an AI model
- Claim errors are routed to the best team member to correct based on past results
- Errors are grouped and prioritized to ensure focus on the most collectible items
The goal with EP is to ensure that the claims are prioritized to optimize reimbursement. This starts with understanding the probability of the claim being reimbursed. An AI model can be designed to assess the likelihood of the claim being reimbursed and the likely reimbursement amount for those expected to be paid. This helps prioritize activities and optimize labor resources. The AI model can also consider important factors such as timely filing dates. If a claim is less likely to be collected than another procedure but is close to its timely filing deadline, it can be escalated. The algorithms can be run nightly to produce a prioritized list of claims with assignments to the specific team member best suited to address each error.
AI can also create a comprehensive list of activities and the order in which those activities should be performed to optimize reimbursement. The result is a prioritized list for each team member indicating which claims should be worked on first and which specific activities need to be accomplished for each claim.
RCM-Focused AI Skills
Diagnostic executives looking to maximize reimbursement and keep the cost of collection low will want to explore how to better leverage data, AI, automation, and analytics across their RCM process. To conclude this series, here is a list of things you should be looking for if you’re interested in implementing AI with a revenue cycle partner.
We recommend that you look for the following skills when evaluating a current or potential RCM partner:
- Deep understanding of healthcare data models and metrics specific to financial and operational workflow
- Can scope and deliver business-critical metrics and indicators
- Experience developing customized and reusable AI models and can integrate data from multiple sources
- Has a proven track record of working with combined clinical and financial analytics
- Can advise about which AI approaches (e.g., statistical, machine learning, natural language processing, and/or generative AI) are best by purpose
- Offer consultative services to identify the most appropriate/useful analytics to achieve a particular goal or address a specific challenge
- Can supplement existing analytics/AI resources on a short-term basis or longer-term engagement
Learn more about leveraging AI in the RCM process by watching the recording of our recent webinar with Dark Daily, “Unlocking RCM Potential: How AI Is Shaping Revenue Cycle Management Workflow.”