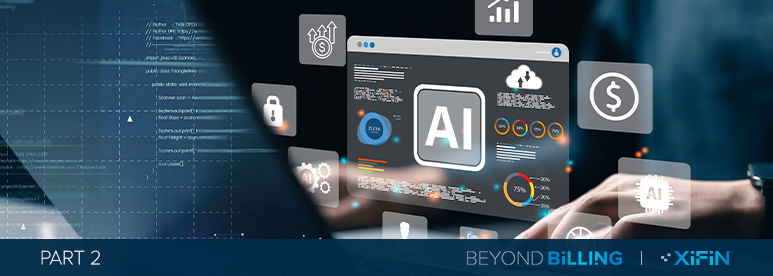
Everything Artificial Intelligence (AI) Starts with Data
January 4, 2024Financial and operational leaders of laboratories, medical device, remote patient monitoring organizations, and other diagnostic providers are under constant pressure to maximize revenue collected amidst constantly changing payor policies and behavior and to do so cost-effectively. This is no easy task. Artificial intelligence (AI) can play several important roles in overcoming these challenges and automating the revenue cycle management (RCM) process.
This is the second in a series of posts addressing how AI is shaping RCM. If you missed our first post, which explores the foundational terms and definitions to understand what AI can do to solve real-world RCM challenges, you can find that post here.
Everything AI-related starts with the data. Without good-quality data, organizations can’t generate AI models that will move a business forward. To build effective AI models, an organization must understand the data landscape, be able to monitor and measure performance and progress, and adjust the activities being driven, as necessary.
Dirty, unstructured data leads to unintelligent AI. AI embodies the old adage, “garbage in, garbage out.” The quality of the AI decision or prediction is entirely based on the quality of historical data it’s seen. If that data is faulty, flawed, or incomplete, it can lead to bad decisions or the inability to predict or make a decision at all. Purposeful data modeling is critical to AI success, and having people and processes that can understand the complicated RCM data and structure it so it can be effectively analyzed is vital to success.
There is a transformation path, illustrated below, that organizations go through to get from having data stored in individual silos to fully embedded AI. If an organization is struggling with aggregating data to build AI models, it’s at stage one. The goal is stage five, where an organization uses AI as a key differentiator and AI drives meaningful activity.
Click to enlarge.
The transformation starts with structuring data with an underlying data approach that keeps it future-ready. It is this foundation that allows organizations to realize the benefits of AI in a cost-effective and efficient way. Only then can automation happen. Having effective AI models that generate strong predictions is only as valuable as the ability to get that feedback into the revenue cycle system effectively. If not, that value is minimal, because the organization must expend quantities of human energy to try to reconfigure or figure out how to act on the AI predictions being generated. Getting the automation fully embedded in the workflow is the key to getting to the full potential of AI in improving the RCM process.
Where Is Your Organization On this AI Transformation Path?
The following descriptions can help diagnostic organizations identify where they are currently on the path toward data, automation, and AI maturity.
Stage 1: Data as Siloed Activity
Limited capacity for internal data sharing. Lack of data strategy/architecture.
Stage 2: Data Ideation
The value of data and automation is recognized and used to develop a data/automation mindset via business vision and strategy.
Stage 3: Building Data/AI Momentum
The value of data/automation has been proven within the organization and use of standardized tools and AI has begun.
Stage 4: Data/AI as a Differentiator
Data/AI methods are well-defined and self-service capabilities are provided. Data and AI uses are expanded out into the connected ecosystem.
Stage 5: Data/AI as Currency
Data and AI are core to business strategy and are applied to drive optimal business outcomes.
Without good-quality data, organizations can’t generate AI models that will move a business forward. To build effective AI models, an organization must understand the data landscape, be able to monitor and measure performance and progress, and adjust the activities being driven, as necessary.
In our next blog in this series, we’ll dive into several specific examples of how AI is automating critical areas within the RCM process, reducing labor and its associated expenses, while improving decision consistency, and accelerating the time to reimbursement.
Until then, you can learn more by watching the recording of our recent webinar with Dark Daily, “Unlocking RCM Potential: How AI Is Shaping Revenue Cycle Management Workflow.”